Stein's method is a powerful technique from probability theory that can be used to bound the distance between probability measures. Although the method was initially designed as a technique for proving central limit theorems, it has recently caught the attention of the computational statistics and machine learning communities, with applications that include generative modelling, variational inference, sampling, control variates, and measuring the quality of Markov chain Monte Carlo algorithms.
This workshop will introduce the tools needed for Stein's method, and then discuss several recent breakthroughs in computational statistics that have resulted from Stein's method.
Call for Posters: There will be a poster session held over lunch for the physical participants – if you are interested in presenting a poster, please contact chris.oates@ncl.ac.uk .
Click Here to register for the In-person event
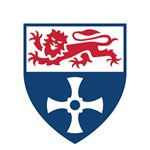
Event sponsored by the School of Mathematics, Statistics & Physics - Newcastle University
Programme:
10:00 – 10:30 Tutorial: Introduction to Stein’s Method. Yvik Swan, Université libre de Bruxelles
10:45 – 11:15Tutorial: Introduction to Kernel Methods. Wenkai Xu, University of Oxford
11:30 – 12:00 Tutorial: Introduction to Bayesian Computation. Chris Nemeth, Lancaster University
Lunch
13:30 – 14:00 Optimal Transport Distance and Stein's Method. Thomas Bonis, Université Gustave Eiffel
14:10 – 14:40 Probabilistic Inference and Learning with Stein's Method. Lester Mackey, Microsoft Research US
Coffee break
15:00 – 15:30 Constrained Variational Inference with Gradient Flow. Qiang Liu, University of Texas at Austin
15:40 – 16:10 Kernelized Stein Discrepancy Tests for Composite Goodness-of-Fit. Tamara Fernandez, Adolfo Ibañez University, Chile
16:20 – end Discussion
Speakers:
- Yvik Swan, Université libre de Bruxelles
- Wenkai Xu, University of Oxford
- Chris Nemeth, Lancaster University.
- Thomas Bonis, Université Paris-Saclay
- Lester Mackey, Microsoft Research, Boston, Massachusetts
- Qiang Liu, University of Texas at Austin
- Tamara Fernandez, Adolfo Ibañez University, Chile
Organisers:Chris Oates and
Gesine Reinert
Organisers group: RSS Applied Probability Section and RSS Computational Statistics and Machine Learning Section